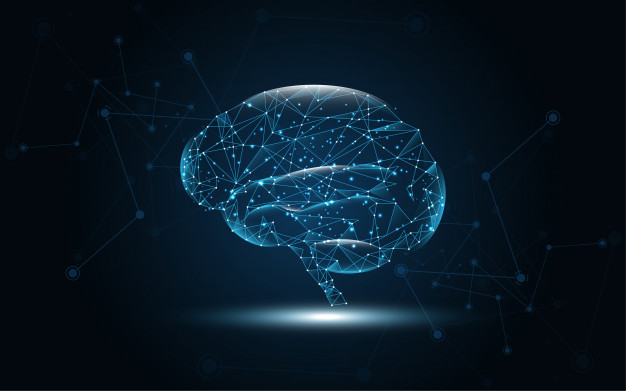
Technology has been making rapid inroads into gathering all sorts of data. This data has remained largely unprocessed, and the bulk of its benefits still hasn’t been tapped into. Quantum computing promises to change that.
These days, cameras, sensors, web crawlers, scanners, and even human operators all collect the big data that often gets thrown away within a definite period of time. If we could only analyze huge datasets in an intelligent and efficient manner, we might be able to identify surprising patterns and regularities of incredible value.
Researchers already use digital tools to do that, but the capabilities of modern computers remain limited. A real breakthrough is possible with the broad introduction of quantum computing. When this happens, many companies, governing bodies and state agencies will wish they had collected the data that seemed too big and messy to handle.
With the recent advancement of artificial intelligence (AI), the potential of quantum computing becomes increasingly clear. Let’s take a closer look at how big data, AI and quantum computing can be combined for unprecedented benefits.
A brief introduction to quantum computing
While regular computers operate on chips using bits, quantum computers use quantum bits or qubits.
Bits work as on/off switches, where the off position is zero and the on position is one. The problem is, the universe is not regulated by simple rules that can be narrowed down to and defined by the two positions, on and off.
Meanwhile, a qubit can be in a superposition, which means it is simultaneously on and off or, in other words, somewhere on the scale between the two positions. Thanks to that, quantum computers can do many more operations in one stretch and thus process more data than even the supercomputers of today.
Although quantum computers are still years away, investors are increasingly interested in this technology.
Founded five years ago, Сalifornian startup PsiQuantum has recently raised $215 million for its photonic quantum computing model. According to its co-founder and CEO Jeremy O’Brien, it will take the company “a handful of years” to present a computer with 1 million qubits.
A boost to artificial intelligence
Artificial intelligence (AI) applications are rooted in big data. Every one of them works by analyzing datasets to find regularities and patterns.
AI techniques have been developing rapidly lately, but their true potential is yet to be unleashed, because it’s limited by the capacities of modern computers. They have already hit the ceiling of their capabilities and fail to process the currently available amounts of data within a reasonable timeframe.
We suggest looking at three key areas of AI as a discipline upon which quantum computing will have a tremendous impact: machine learning, predictive analytics, and natural language processing.
Machine learning
Machine learning (ML) algorithms use sample data to automatically train on. How much data can be processed for this purpose depends on the computing power available to you.
Scientists define quantum machine learning (QML) as an interdisciplinary research area that studies the use of ML algorithms executed on a quantum computer. But there is another scientific approach that suggests looking at qubit-powered AI as “quantum computational intelligence”.
The progress of this technology has been slow but traceable. Quantum programming languages first emerged in the late 1990s. Today, you can tap quantum machine learning with PennyLane, a cross-platform Python library and software framework, Google’s framework TensorFlow Quantum, and PyTorch.
Here is one example: Deep learning neural networks (DLNN) is an ML technique that requires such large amounts of data that it did not seem feasible until the rise of cloud computing. As qubits offer even more number-crunching power, the capabilities of DLNNs will increase alongside the value of big data.
Predictive analytics
AI can analyze data like historical facts and current circumstances to identify patterns and predict events based on these patterns, allowing you to prevent bottlenecks and use your resources wisely. With quantum computing power, you will be able to process more of such data and accelerate the detection of relevant, high-quality information to use for predictions.
In the past, the development of predictive models was hampered by datasets that were too small due to the cost of collecting, storing and searching data. Today, you face a completely different challenge: the volumes of currently available data can overwhelm a predictive model.
As data volumes grow, so do the numbers of decision variables and predicting factors. The capabilities of quantum computing promise to help build more scalable predictive models that can deal with the huge loads of data and add as many variables to the equation as possible without slowing down essential processes.
This, in turn, promises much more specific and useful insights than currently available.
Whether you are looking for an efficient flight scheduling model, an informed inventory decision-making process, streamlined delivery routing, or other workflow optimization opportunities, predictive analytics powered by quantum computing is what you should be looking at applying.
Therefore, the data you are collecting today will determine your success tomorrow. For a more specific example, Steve Rietberg, Senior Director Analyst at Gartner, suggests that businesses should prepare for the advancement of predictive analytics by “tracking key buyer activities in their CRM” to “build up a bank of buyer behavior and outcome data”.
Natural language processing
The quality of interaction between humans and computers is increasingly important today, as businesses keep improving their processes with chatbots and other advanced automation techniques. Natural language processing (NLP), one of the most hyped applications of AI, is responsible for that.
The key technique in NLP is deep learning, which is a machine learning method. It implies training neural networks on a vast variety of datasets, such as images, text, and sound.
The training data sets should be big enough to avoid overfitting: with insufficient data, a deep neural network tends to memorize the training set, which results in poor performance on the test set.
During the Frontiers of NLP session at the Deep Learning Indaba 2018, a machine learning expert, Bernardt Duvenhage, argued that languages have universal commonalities. These commonalities could be leveraged in creating and training a universal language model, but there are two road bumps: enough data and enough compute.
If the lack of data remains a nuisance for low-resource languages, the compute is the main concern in dealing with languages that come with enough data. There already have been successful attempts, such as OpenAI Five, that demonstrate how much better current neural lingual models would perform if they are backed by more compute.
In April 2020, Cambridge Quantum Computing revealed some of the details of the first-ever quantum-powered execution of NLP.
The scientists claim to have been able to “open up an entirely new realm of possible applications by translating grammatical sentences into quantum circuits, and then implementing the resulting programs on a quantum computer and actually performing question-answering”.
Conclusion
At this point, you must have a good idea of the impressive opportunities for big data use with quantum-powered AI.
Take a look at your business processes. Is there data that you think is not worth collecting because it seems unmanageable? Can you see how you could use it in the future, with the advancement of quantum computing, to ensure an ultimate competitive advantage for your enterprise?
If this still seems too difficult to get a sense of, don’t fret. Consult Intetics Inc. and we will help you prepare for the not-so-distant quantum future. We can guide you through the next level of digital transformation and establish an intelligent, cost-efficient data collection system within your organization.