According to Deloitte’s report on Conversational AI, 50% of customers would immediately proceed with a Conversational AI chatbot, while 90% would use CAI when waiting. Unhappy customers cost businesses USD 537 trillion yearly, while business inefficiency losses remain uncalculated.
Conversational AI is a highly lucrative technology for enterprises, helping businesses prevent customer attrition, enhance experience, and become more profitable.
While AI-powered chatbots and virtual assistants are the most popular forms of conversational AI, many other use cases exist across industries.
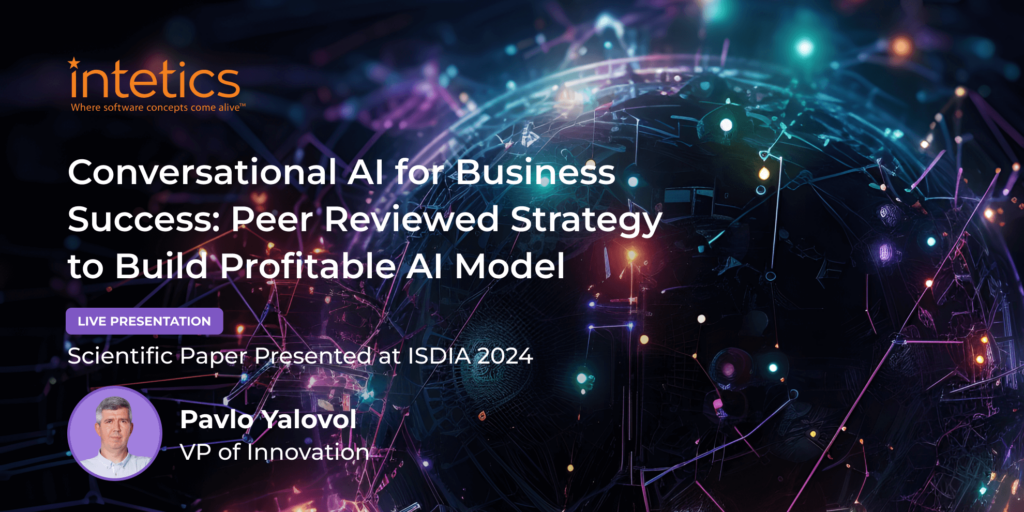
Discover business applications, benefits, and strategies for implementing Conversational AI within an organization, peer-reviewed by the global academic community.
Conversational AI for Business Success: How to Amplify Artificial Intelligence
On January 4, Pavlo Yalovol, Intetics VP of Innovation, presented the scientific paper ‘Conversational AI for Business Success: How to Amplify Artificial Intelligence’ written by Boris Kontsevoi, Intetics CEO and President, at the virtual stage of the eighth edition of International Conference on Information System Design and Intelligent Applications (ISDIA).
The ISDIA 2024 conference brought together researchers, scientists, engineers, students, and industry practitioners to exchange theories, methodologies, new ideas, experiences, products, and applications in all areas of intelligent computing methodologies. Participants explored how AI, ML, and other innovative technologies enhance and safeguard our interconnected world.
Get a glimpse of the key presentation insights, and feel free to access the full paper by reaching out.
AI in Action: Advancements, Capabilities, and Transformative Benefits
Conversational AI and virtual voice assistants with natural language processing (NLP) and machine learning under the hood have emerged to simplify daily routines by handling tedious, time-consuming, or complex tasks. NLP deciphers human language, while ML enables learning from data, making these technologies proficient in human-like interactions.
The adoption of AI experienced a 10% surge from 2021 to 2023, attributed to the proactive efforts of IT professionals. Projections indicate a substantial annual increase of 38% in the global AI market value from 2022 to 2030.
Recent research by McKinsey highlights the financial advantages of incorporating conversational AI, positioning companies with a competitive edge, and driving heightened investments in AI development.
Conversational AI’s Potential across Industries
AI-driven chatbots and virtual assistants enable seamless conversations, automate mundane tasks, and gather valuable customer insights across Healthcare, Finance, E-commerce, Education, and other industries:
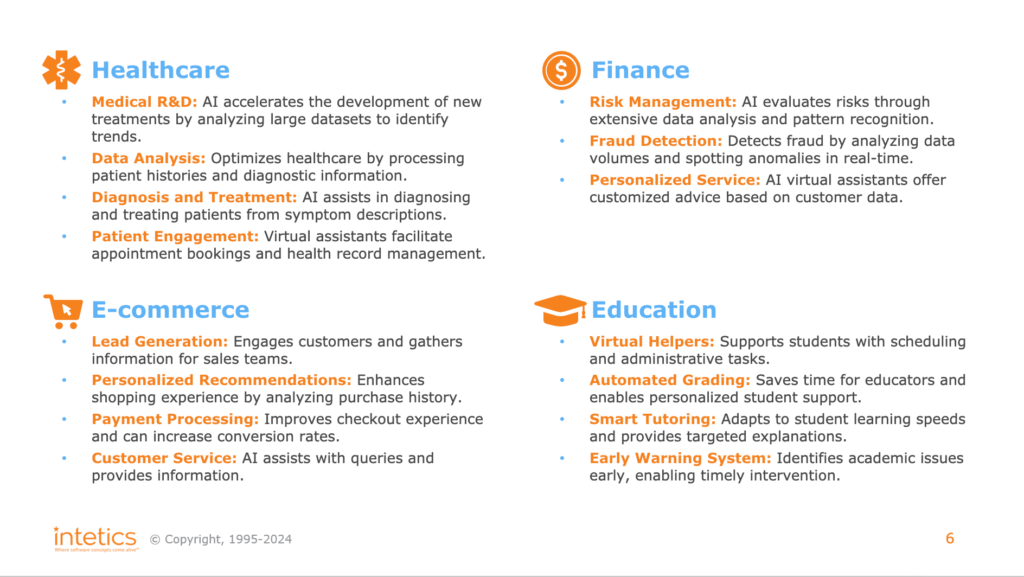
Featured Case Study: AI-Powered Virtual Nurse Helps Over 100,000 Patients Worldwide to Monitor Their Health Daily
Advancements in NLP: Models & Algorithms
Recurrent neural networks for generating word sequences enable tasks like text prediction, handwriting recognition, and speech recognition. Explore the newer NLP models, which can answer intricate research questions and process information across multiple languages.
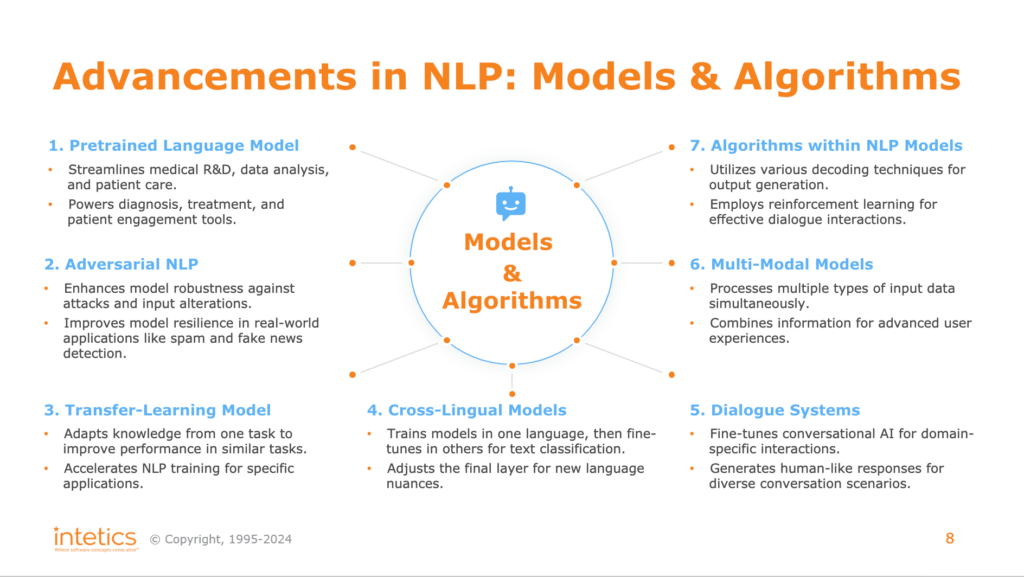
Different Types of NLP Models
There are four key neural network architectures:
- Feedforward Neural Networks (FNNs): Process data unidirectionally from input to output. Suited for tasks without sequential/temporal dependencies. Applied in classification and regression.
- Convolutional Neural Networks (CNNs): Ideal for grid-structured data like images. Use convolutional layers to detect patterns. CNNs are used in image recognition and object detection.
- Generative Adversarial Networks (GANs): Composed of a generator and discriminator network. The generator creates data; the discriminator differentiates from accurate data. GANs are employed in image generation and data augmentation.
- Recurrent Neural Networks (RNNs): Designed for sequential data processing. Feedback loops allow the learning of patterns in sequences. RNNs are applied in language processing and time series analysis. Challenges like vanishing gradients are addressed by LSTM and GRU variants.
The Ethical and Reliability Challenges of AI
Discover core challenges faced by conversational AI that businesses should be aware of:
- Understanding Context: AI may struggle with broad contexts and subtle nuances, leading to misinterpretations.
- Data Bias Risks: Reliance on incomplete or biased training data can lead to skewed and unfair interaction outcomes.
- Human Oversight Necessity: Expert review is essential to mitigate risks of unexpected consequences from AI autonomy.
- Vulnerabilities to Exploitation. AI systems can be exploited for disinformation and propaganda.
- Consumer Trust. McKinsey survey reveals high consumer trust in AI, often surpassing human expertise.
- Realism and Validation. AI should not be the sole decision-making tool; rigorous human testing and validation are crucial.
NLP API Options: A Look into Leading Choices
There are various APIs (Application Programming Interfaces), which encompass a range of functionalities, including sentiment analysis, entity recognition, text categorization, language detection, text generation, and more.
Therefore, when selecting an API, several key factors should be taken into consideration: customization and control, complexity of use case, platform compatibility, and integration ease. Some of the prominent NLP APIs are OpenAI GPT, Google Cloud Natural Language API, Google Dialogflow, Amazon Comprehend & Lex, IBM Watson NLU & Assistant, Microsoft Azure Cognitive Services, Wit.ai, spaCy, Algorithmia, and others.
Key Steps to Developing a Successful Conversational AI Business Model
Formulating a successful conversational AI business model implies these crucial steps:
- Defining the niche. Identify a specific domain where conversational AI solutions can provide value. The niche should align with the expertise of the business and cater to a genuine market need.
- Creating a high-quality product. The product must possess natural communication, complex query understanding, accurate responses, and scalability.
- Leveraging NLP tools. Integrating NLP enhances the AI’s ability to engage with users intuitively.
- Integration with existing systems. Seamless integration with established business systems (CRM, ERP, help desk software, etc.) bolsters the AI’s capabilities and elevates the overall customer experience.
- Developing a go-to-market strategy. This strategy should encompass the following elements: target audience definition, needs and preferences analysis, and marketing plan.
- Monitoring and optimization. Utilize analytics tools to glean insights from customer inquiries and employ these insights to drive continuous improvements that enhance system performance.
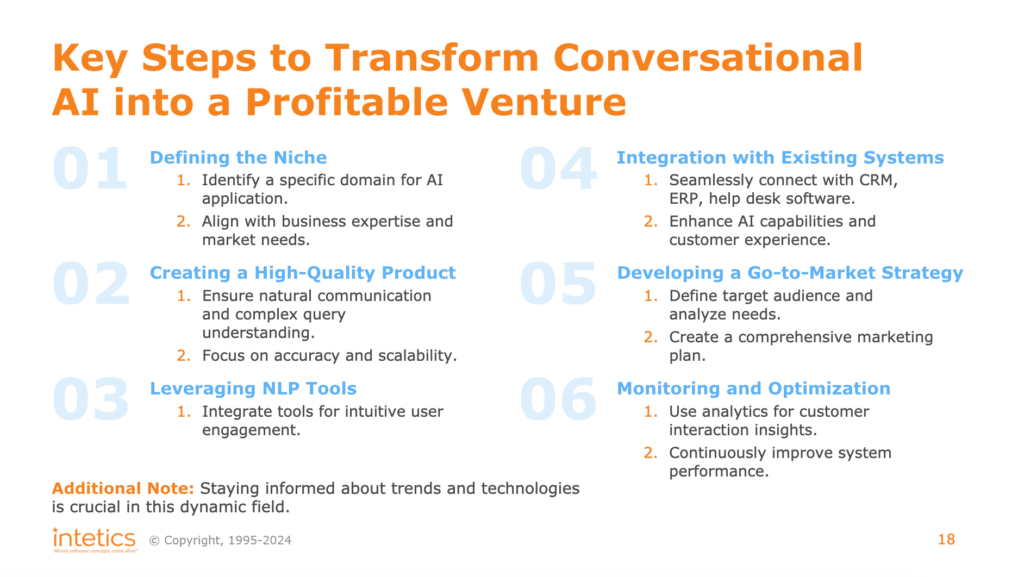
Conversational AI can enhance lives when used appropriately and aligned with user expectations. Here are a few examples and numbers:
- Business Process Optimization. Up to 25% cost reduction and 30% productivity growth with record management, task management, auto-scheduling, email filtering, and fast onboarding and training powered by CAI.
- Enhanced Decision-Making. Up to 95% of information accuracy thanks to easy report generation, advanced analytics, and smart recommendations.
- Advanced Customer Interactions. Customer satisfaction increases by up to 50% with service/product recommendations, intelligent comparison, and a robust sales funnel.
Successful implementation demands meticulous planning, experimentation, and continuous monitoring to achieve desired outcomes.
Are you considering starting to plan and implement conversational AI technologies for your niche? Get started with advanced approaches and industry solutions now.